Visually Grounded Interaction and Language (ViGIL)
NeurIPS 2019 Workshop, Vancouver, Canada
Introduction
The dominant paradigm in modern natural language understanding is learning statistical language models from text-only corpora. This approach is founded on a distributional notion of semantics, i.e. that the "meaning" of a word is based only on its relationship to other words. While effective for many applications, methods in this family suffer from limited semantic understanding, as they miss learning from the multimodal and interactive environment in which communication often takes place - the symbols of language thus are not grounded in anything concrete. The symbol grounding problem first highlighted this limitation, that "meaningless symbols (i.e.) words cannot be grounded in anything but other meaningless symbols" [1].
On the other hand, humans acquire language by communicating about and interacting within a rich, perceptual environment. This behavior provides the necessary grounding for symbols, i.e. to concrete objects or concepts (i.e. physical or psychological). Thus, recent work has aimed to bridge vision, interactive learning, and natural language understanding through language learning tasks based on natural images (ReferIt [2], Visual Question Answering [3-6], Visual Dialog [7-10], Captioning [11, 32-35], Visual-Audio Correspondence [30]) or through embodied agents performing interactive tasks [12-29] in physically simulated environments (DeepMind Lab [12], Baidu XWorld [13], Habitat [14], StreetLearn [18], AI2-THOR [21], House3D [22], Matterport3D [23], GIBSON [27], MINOS [28]), often drawing on the recent successes of deep learning and reinforcement learning. We believe this line of research poses a promising, long-term solution to the grounding problem faced by current, popular language understanding models.
While machine learning research exploring visually-grounded language learning may be in its earlier stages, it may be possible to draw insights from the rich research literature on human language acquisition. In neuroscience, recent progress in fMRI technology has enabled better understanding of the interaction between language, vision and other modalities [17,18] suggesting that the brains share neural representations of concepts across vision and language. In concurrent work, developmental cognitive scientists have argued that word acquisition in children is closely linked to them learning the underlying physical concepts in the real world [15, 31], and that they generalize surprisingly well at this from sparse evidence [36].
This workshop thus aims to gather people from various backgrounds - machine learning, computer vision, natural language processing, neuroscience, cognitive science, psychology, and philosophy - to share and debate their perspectives on why grounding may (or may not) be important in building machines that truly understand natural language.
Important Dates
Paper Submission Deadline | September 18, 2019 (11:59 PM Pacific time) |
Decision Notifications | |
Workshop | December 13, 2019 |
Call for Papers
We invite high-quality paper submissions on the following topics:
- language acquisition or learning through interactions
- image/video captioning, visual dialogues, visual question-answering, and other visually grounded language challenges
- reasoning in language and vision
- transfer learning in language and vision tasks
- audiovisual scene understanding and generation
- navigation and question answering in virtual worlds with natural-language instructions
- original multimodal works that can be extended to vision, language or interaction
- human-machine interaction with vision and language
- understanding and modeling the relationship between language and vision in humans semantic systems and modeling of natural language and visual stimuli representations in the human brain
- epistemology and research reflexions about language grounding, human embodiment and other related topics
- visual and linguistic cognition in infancy and/or adults
Submission
Please upload submissions at: cmt3.research.microsoft.com/VIGIL2019
Accepted papers will be presented during joint poster sessions, with exceptional submissions selected for spotlight oral presentations. Accepted papers will be made publicly available as non-archival reports, allowing future submissions to archival conferences or journals.
Submissions should be up to 4 pages excluding references, acknowledgements, and supplementary material, and should follow NeurIPS format. The CMT-based review process will be double-blind to avoid potential conflicts of interests.
We welcome published papers from *non-ML* conferences that are within the scope of the workshop (without re-formatting). These specific papers do not have to be anonymous. They are eligible for poster sessions and will only have a very light review process.
A limited pool of NeurIPS registrations might be available for accepted papers.
In case of any issues, feel free to email the workshop organizers at: vigilworkshop@gmail.com.
D-Day Workshop Information
Posters will be taped to the wall (we will provide tape).
Please make sure they are printed on lightweight paper without lamination
and no larger than 36 x 48 inches or 90 x 122 cm in portrait orientation.
Schedule
08:20 AM - 08:30 AM | Opening remarks [Video] |
08:30 AM - 09:10 AM | Invited talk: Jason Baldridge [Video] |
09:10 AM - 09:50 AM | Invited talk: Jesse Thomason [Video] |
09:50 AM - 10:30 AM | Coffee break |
10:30 AM - 10:50 AM |
Spotlights
[Video]
|
10:50 AM - 11:30 AM | Invited talk: Jay McClelland [Video] |
11:30 AM - 12:10 PM | Invited talk: Louis-Philippe Morency [Video] |
12:10 PM - 01:50 PM | Poster session + Lunch |
01:50 PM - 02:30 PM | Invited talk: Lisa Anne Hendricks [Video] |
02:30 PM - 03:10 PM | Invited talk: Linda Smith [Video] |
03:10 PM - 04:00 PM | Poster session + Coffee break |
04:00 PM - 04:40 PM | Invited talk: Timothy Lillicrap [Video] |
04:40 PM - 05:20 PM | Invited talk: Josh Tenenbaum (presented by Jiayuan Mao) [Video] |
05:20 PM - 06:00 PM | Panel Discussion [Video] |
06:00 PM - 06:10 PM | Closing remarks |
Invited Speakers
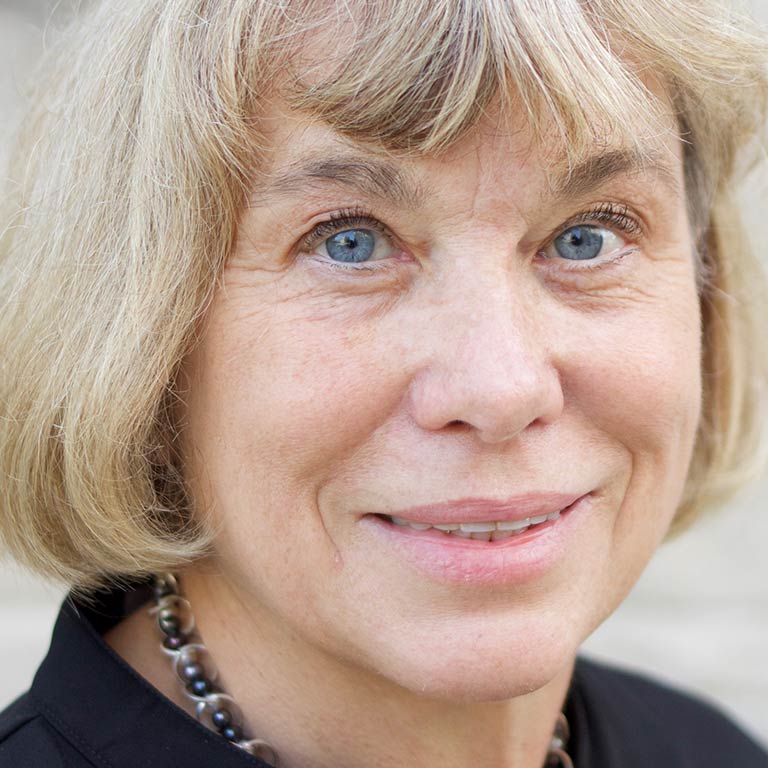
Linda Smith is a Distinguished Professor, Psychological and Brain Sciences at Indiana University. Her recent work at the intersection of cognitive development and machine learning focuses specifically on the statistics of infants' visual experience and how it affects concept and word learning. [Webpage]
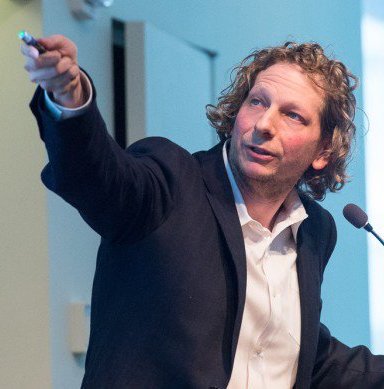
Josh Tenenbaum is a Professor in Computational Cognitive Science at MIT. His work studies learning and reasoning in humans and machines, with the twin goals of understanding human intelligence in computational terms and bringing artificial intelligence closer to human-level capacities. [Webpage]
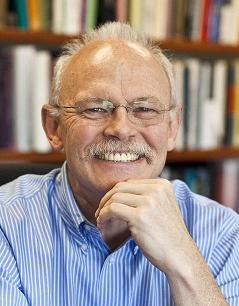
Jay McClelland is a Professor in the Psychology Department and Director of the Center for Mind, Brain and Computation at Stanford University. His research spans a broad range of topics in cognitive science and cognitive neuroscience, including perception and perceptual decision making; learning and memory; language and reading; semantic and mathematical cognition; and cognitive development. [Webpage]
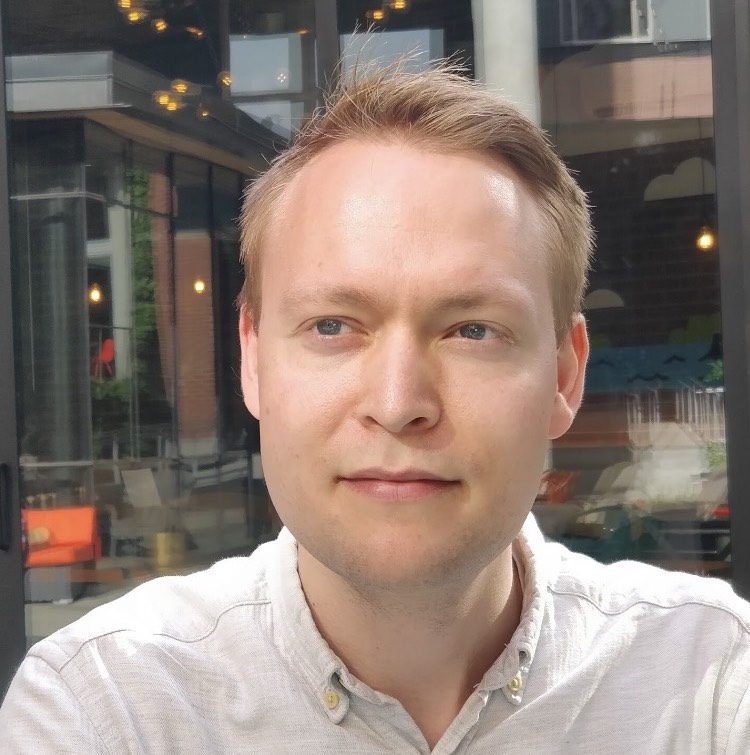
Jesse Thomason is a postdoctoral researcher at the University of Washington. His research focuses on language grounding and natural language processing applications for robotics, including how dialog with humans can facilitate both robot task execution and learning. [Webpage]
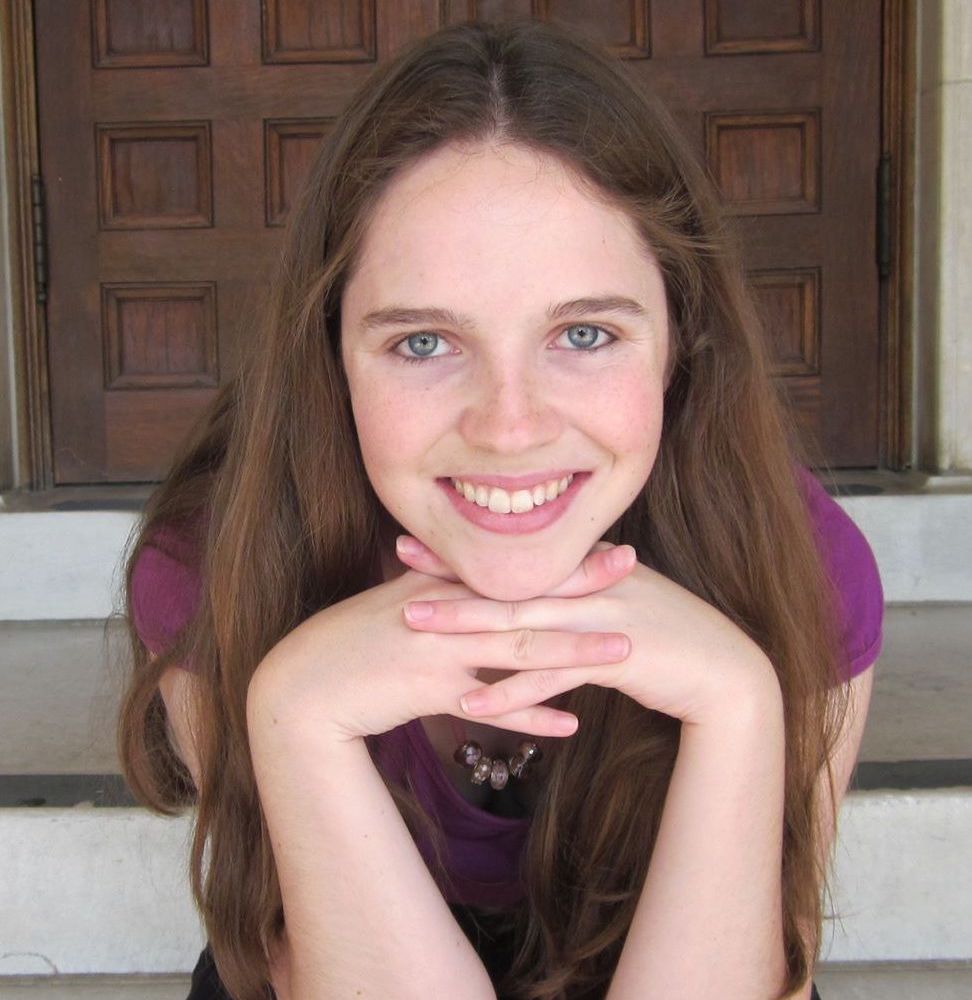
Lisa Anne Hendricks is a research scientist at DeepMind (previously a PhD student in Computer Vision at UC Berkeley). Her work focuses on building systems which can express information about visual content using natural language and retrieve visual information given natural language. [Webpage]
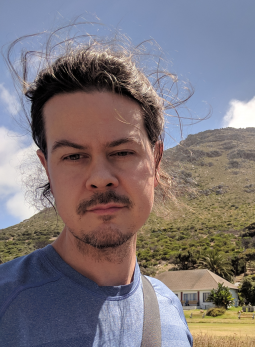
Timothy Lillicrap is a research scientist at DeepMind. His research focuses on machine learning and statistics for optimal control and decision making, as well as using these mathematical frameworks to understand how the brain learns. He has developed algorithms and approaches for exploiting deep neural networks in the context of reinforcement learning, and recurrent memory architectures for one-shot learning. [Webpage]
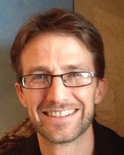
Jason Baldridge is a research scientist at Google. His research focuses on the theoretical and applied aspects of computational linguistics -- from formal and computational models of syntax to machine learning for natural language processing and geotemporal grounding of natural language. [Webpage]
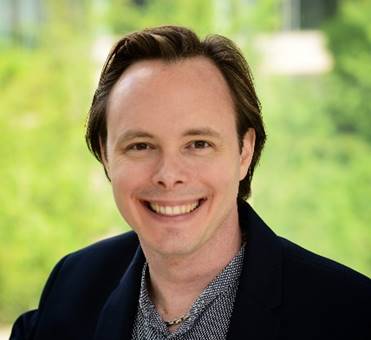
Louis-Philippe Morency is an Associate Professor at the Language Technology Institute at Carnegie Mellon University. His research focuses on building the computational foundations to enable computers with the abilities to analyze, recognize and predict subtle human communicative behaviors during social interactions. [Webpage]
Accepted Papers
-
Help, Anna! Visual Navigation with Natural Multimodal Assistance via Retrospective Curiosity-Encouraging Imitation Learning
-
What is needed for simple spatial language capabilities in VQA?
-
Learning from Observation-Only Demonstration for Task-Oriented Language Grounding via Self-Examination
-
Not All Actions Are Equal: Learning to Stop in Language-Grounded Urban Navigation
-
Hidden State Guidance: Improving Image Captioning Using an Image Conditioned Autoencoder
-
Situated Grounding Facilitates Multimodal Concept Learning for AI
-
VideoNavQA: Bridging the Gap between Visual and Embodied Question Answering
-
Induced Attention Invariance: Defending VQA Models against Adversarial Attacks
-
Natural Language Grounded Multitask Navigation
-
Contextual Grounding of Natural Language Entities in Images
-
Visual Dialog for Radiology: Data Curation and FirstSteps
-
Multimodal Generative Learning Utilizing Jensen-Shannon-Divergence
-
Learning Question-Guided Video Representation for Multi-Turn Video Question Answering
-
Structural and functional learning for learning language use
-
Community size effect in artificial learning systems
-
CLOSURE: Assessing Systematic Generalization of CLEVR Models
-
A Comprehensive Analysis of Semantic Compositionality in Text-to-Image Generation
-
Recurrent Instance Segmentation using Sequences of Referring Expressions
-
Visually Grounded Video Reasoning in Selective Attention Memory
-
Modulated Self-attention Convolutional Network for VQA
-
General Evaluation for Instruction Conditioned Navigation using Dynamic Time Warping
-
A Simple Baseline for Visual Commonsense Reasoning
-
Language Grounding through Social Interactions and Curiosity-Driven Multi-Goal Learning
-
Deep compositional robotic planners that follow natural language commands
-
Can adversarial training learn image captioning ?
-
Leveraging Topics and Audio Features with Multimodal Attention for Audio Visual Scene-Aware Dialog
-
Supervised Multimodal Bitransformers for Classifying Images and Text
-
Shaping Visual Representations with Language for Few-shot Classification
-
Self-Educated Language Agent with Hindsight Experience Replay for Instruction Following
-
Analyzing Compositionality in Visual Question Answering
-
On Agreements in Visual Understanding
-
A perspective on multi-agent communication for information fusion
-
Cross-Modal Mapping for Generalized Zero-Shot Learning by Soft-Labeling
-
Learning Language from Vision
-
Commonsense and Semantic-Guided Navigation through Language in Embodied Environment
Organizers
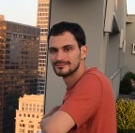
University of Lille, Inria | DeepMind
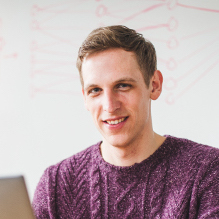
University of Montreal
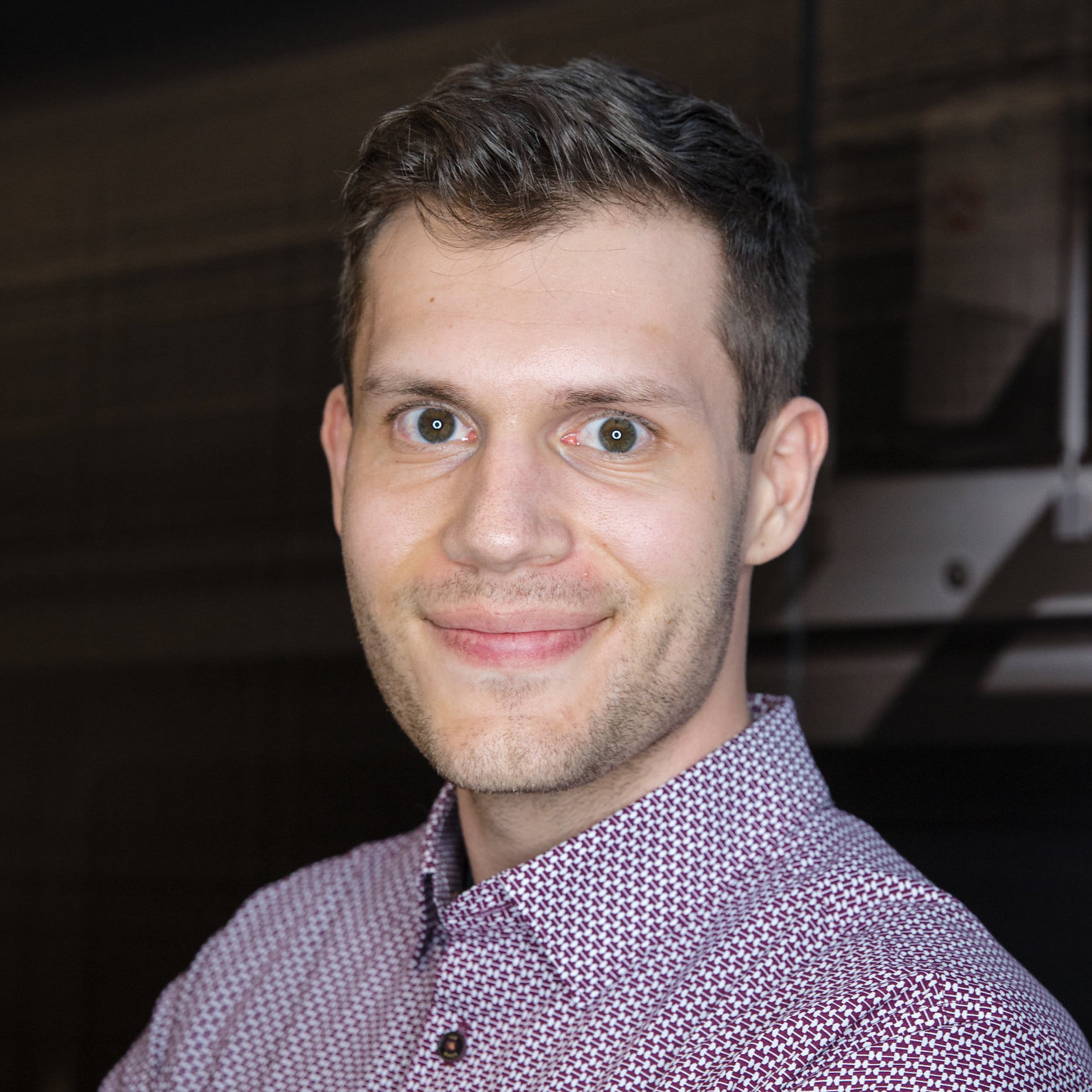
Georgia Tech
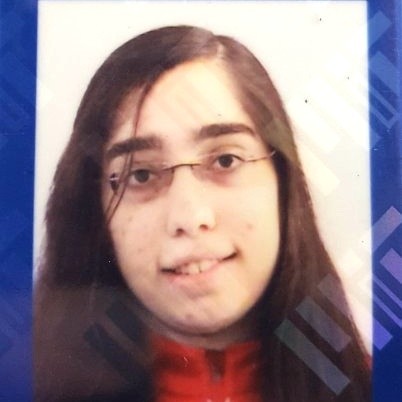
Stanford University
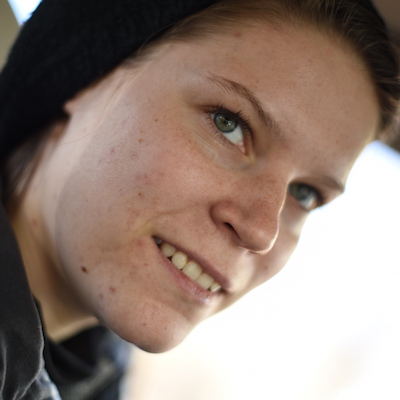
Cornell University
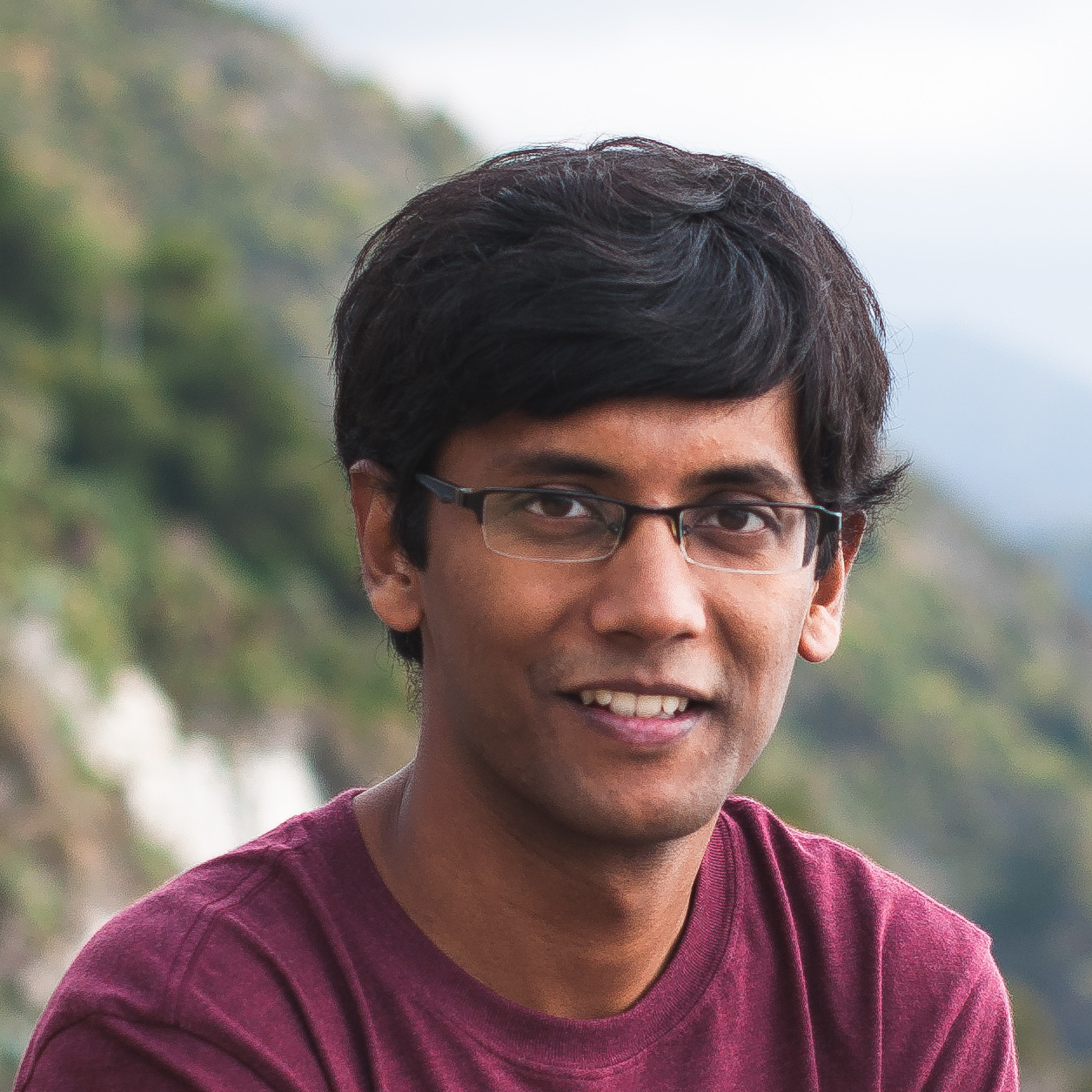
Georgia Tech
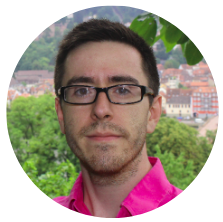
Oregon State University
Scientific Committee
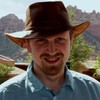
DeepMind
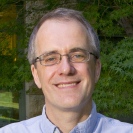
Stanford University
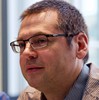
University of Montreal
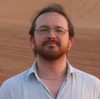
Google Brain
Program Committee
- Aishwarya Agrawal
- Cătălina Cangea
- Volkan Cirik
- Meera Hahn
- Ethan Perez
- Rowan Zellers
- Ryan Benmalek
- Luca Celotti
- Daniel Fried
- Arjun Majumdar
- Hao Tan
Previous sessions
Sponsors
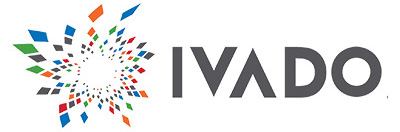
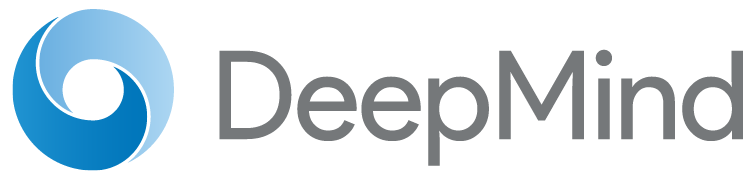
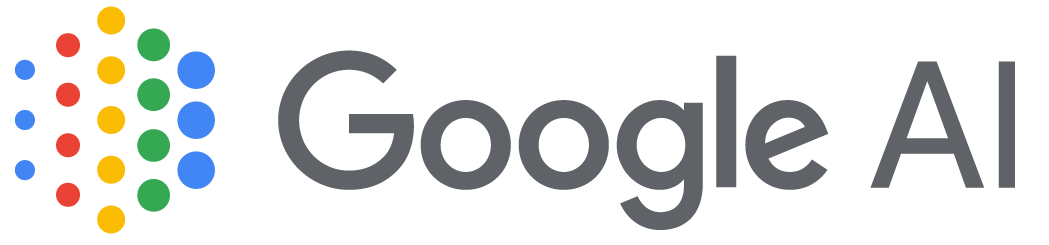
References
- Stevan Harnad. "The symbol grounding problem." CNLS, 1989.
- Sahar Kazemzadeh et al. "ReferItGame: Referring to Objects in Photographs of Natural Scenes." EMNLP, 2014.
- Stanislaw Antol et al. "VQA: Visual question answering." ICCV, 2015.
- Mateusz Malinowski et al. "Ask Your Neurons: A Neural-based Approach to Answering Questions about Images." ICCV, 2015.
- Mateusz Malinowski et al. "A Multi-World Approach to Question Answering about Real-World Scenes based on Uncertain Input." NIPS, 2014.
- Geman Donald, et al. "Visual Turing test for computer vision systems." PNAS, 2015.
- Abhishek Das et al. "Visual dialog." CVPR, 2017.
- Harm de Vries et al. "GuessWhat?! Visual object discovery through multi-modal dialogue." CVPR, 2017.
- Abhishek Das et al. "Learning Cooperative Visual Dialog Agents with Deep Reinforcement Learning." ICCV, 2017.
- Huda Alamri et al. "Audio-Visual Scene-Aware Dialog" CVPR, 2019.
- Anna Rohrbach et al. "Generating Descriptions with Grounded and Co-Referenced People." CVPR, 2017.
- Charles Beattie et al. Deepmind Lab. arXiv, 2016.
- Haonan Yu et al. "Guided Feature Transformation (GFT): A Neural Language Grounding Module for Embodied Agents." arXiv, 2018.
- Habitat: A Platform for Embodied AI Research. 2019.
- Alison Gopnik et al. "Semantic and cognitive development in 15- to 21-month-old children." Journal of Child Language, 1984.
- Karl Moritz Hermann et al. "Grounded Language Learning in a Simulated 3D World." arXiv, 2017.
- Alexander G. Huth et al. "Natural speech reveals the semantic maps that tile human cerebral cortex." Nature, 2016.
- Alexander G. Huth et al. "Decoding the semantic content of natural movies from human brain activity." Frontiers in systems neuroscience, 2016.
- Piotr Mirowski et al. "Learning to Navigate in Cities Without a Map." NeurIPS, 2018.
- Karl Moritz Hermann et al. "Learning to Follow Directions in StreetView." arXiv, 2019.
- E Kolve et al. "AI2-THOR: An Interactive 3D Environment for Visual AI." arXiv, 2017.
- Yi Wu et al. "House3D: A Rich and Realistic 3D Environment." arXiv, 2017.
- Angel Chang et al. "Matterport3D: Learning from RGB-D Data in Indoor Environments." arXiv, 2017.
- Abhishek Das et al. "Embodied Question Answering." CVPR, 2018.
- Peter Anderson et al. "Vision-and-Language Navigation: Interpreting visually-grounded navigation instructions in real environments." CVPR, 2018.
- Xin Wang et al. "Reinforced Cross-Modal Matching and Self-Supervised Imitation Learning for Vision-Language Navigation." CVPR, 2019.
- Fei Xia et al. "Gibson Env: Real-World Perception for Embodied Agents." CVPR, 2018.
- Manolis Savva et al. "MINOS: Multimodal indoor simulator for navigation in complex environments." arXiv, 2017.
- Daniel Gordon et al. "IQA: Visual Question Answering in Interactive Environments." CVPR, 2018.
- Relja Arandjelovic et al. "Look, Listen and Learn." ICCV, 2017.
- Jessica Montag et al. "Quantity and Diversity: Simulating Early Word Learning Environments." Cognitive Science, 2018.
- Oriol Vinyals et al. "Show and Tell: A Neural Image Caption Generator." CVPR, 2015.
- Andrej Karpathy et al. "Deep Visual-Semantic Alignments for Generating Image Descriptions." CVPR, 2015.
- Jeff Donahue et al. "Long-Term Recurrent Convolutional Networks for Visual Recognition and Description." CVPR, 2015.
- Lisa Anne Hendricks et al. "Deep Compositional Captioning: Describing Novel Object Categories Without Paired Training Data." CVPR, 2016.
- Brendan Lake et al. "Human-level concept learning through probabilistic program induction." Science, 2015.